Histogram Analysis
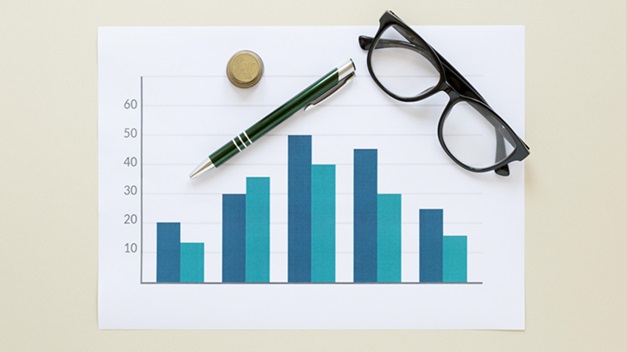
1. Introduction to Histogram Analysis in Pharma and Biopharma
In the pharmaceutical and biopharmaceutical industries, data analysis plays a critical role in drug discovery, clinical trials, manufacturing, and regulatory compliance. One of the most fundamental and widely used methods of data visualization and analysis is the histogram, which helps to illustrate data distributions and provide insights into underlying patterns, trends, and anomalies.
Histograms offer a visual representation of the frequency distribution of a dataset, breaking data into specific intervals or "bins." This graphical representation is essential for identifying outliers, understanding variability, and assessing the normalcy of data. In the pharmaceutical and biopharma sectors, where massive datasets are generated from research studies, clinical trials, manufacturing, and quality control, histogram analysis becomes indispensable.
Histograms enable stakeholders to make informed decisions by visualizing crucial aspects of data such as drug efficacy, patient response variability, manufacturing consistency, and safety issues. As the industry continues to evolve, the ability to quickly interpret data using tools like histograms is critical to ensure product safety, efficacy, and compliance with regulatory requirements.
2. Basic Concepts of Histogram Analysis
A histogram is a type of bar graph used to represent the distribution of a dataset. The data is divided into intervals, known as bins, and the height of each bar reflects the number of data points that fall within that specific range. This provides a clear visual representation of how the data is spread across a continuum, enabling the identification of key patterns.
The major components of a histogram include:
- Bins: These represent the intervals into which the data is divided. For example, in a drug trial, the bins could represent ranges of patient ages or different dosage levels.
- Frequency: The vertical axis of a histogram indicates the frequency, or how often data points fall within each bin. This shows the concentration of data in different ranges.
- Distribution: A histogram provides insight into the overall distribution of the data, whether it’s normal, skewed, bimodal, or showing some other pattern. This helps in understanding whether data follows expected trends or if there are deviations.
In the pharmaceutical and biopharma industries, histograms help visualize the distribution of critical datasets like compound efficacy in drug discovery, patient outcomes in clinical trials, or process variables in manufacturing. By providing a simple yet powerful way to analyze complex data, histograms serve as a foundational tool for researchers, clinicians, and quality control experts.
3. Applications of Histogram Analysis in Drug Discovery
Drug discovery is one of the most data-intensive phases in the pharmaceutical and biopharma industries, particularly when it involves high-throughput screening (HTS), which tests large libraries of compounds for biological activity. Histogram analysis plays an essential role in evaluating the results of these screenings.
- Analyzing HTS Data: In HTS, millions of compounds are tested for their biological activity, generating massive datasets. A histogram can help visualize the distribution of compound activity, allowing researchers to quickly assess the spread of activity levels. Compounds can be grouped into bins representing different levels of activity, helping scientists identify the most promising drug candidates.
- Compound Distribution and Potency Analysis: A histogram can also represent the distribution of compound potency. This allows researchers to observe how many compounds exhibit high potency versus those that show limited or no activity. Understanding the potency distribution is critical when making decisions about which compounds to prioritize for further study.
- Assay Variability and Hit Selection: In drug discovery, assay variability can impact how reliable the data is. By plotting assay results in a histogram, researchers can easily detect variability and identify outliers. This is particularly useful in hit selection, where histogram analysis helps determine which compounds are genuine hits (showing consistent, reproducible results) and which may be false positives due to assay noise.
Histograms provide a quick and effective method to sift through large volumes of data, helping researchers visualize compound activity and potency while ensuring that only the most promising candidates move forward in the drug discovery pipeline.
4. Role of Histogram Analysis in Clinical Trials
Clinical trials are a critical phase of drug development, and understanding patient responses, safety, and efficacy data is essential for moving a product through the regulatory approval process. Histogram analysis is particularly valuable in understanding data distribution in clinical trials, offering clear visualizations of how patients respond to treatment, the frequency of adverse events, and the overall trial outcome.
- Patient Data Distribution: One common use of histograms in clinical trials is to represent the distribution of patient demographics such as age, gender, comorbidities, or treatment history. A histogram can show, for example, how the age distribution of trial participants is spread out, which is important for understanding the trial's applicability to the target patient population.
- Adverse Event Frequency and Distribution: During clinical trials, adverse events (AEs) are carefully monitored to ensure drug safety. A histogram can be used to visualize the frequency of various AEs across different patient groups, dosages, or time periods. This helps researchers quickly identify if certain AEs are more common in specific subgroups or at particular dosages, leading to better risk management strategies.
- Understanding Treatment Response Variability: Not all patients respond to treatment in the same way. Histograms can be used to visualize the variability in treatment responses, showing how many patients experienced a full response, partial response, or no response at all. This helps in identifying whether the treatment is effective across a broad population or if its efficacy is limited to a subset of patients.
For example, a bimodal distribution in a histogram may indicate that a drug is effective for two distinct subpopulations of patients, suggesting the need for further investigation or even development of personalized treatment approaches.
By providing clear visualizations of clinical trial data, histograms help researchers and regulators make informed decisions about drug efficacy, safety, and future trial designs.
5. Histograms in Quality Control and Manufacturing
In pharmaceutical and biopharma manufacturing, ensuring the consistency and quality of products is paramount. Histogram analysis plays a critical role in process control, helping manufacturers monitor key parameters and identify deviations that could lead to production failures or product recalls.
- Process Control and Batch Consistency: In large-scale drug production, consistency between batches is vital to ensure that each product meets the same standards of quality and efficacy. Histograms are used to monitor key production parameters such as temperature, pH, concentration, and yield. By plotting these variables over time, manufacturers can identify trends or outliers that may indicate problems in the production process.
- Monitoring Production Parameters and Outcomes: Each stage of the manufacturing process can be analyzed using histograms to visualize the distribution of critical quality attributes (CQAs). For instance, histograms can show the variation in the size of nanoparticles in a drug formulation or the distribution of active ingredients within a tablet. By assessing whether these distributions are within acceptable ranges, manufacturers can ensure product quality.
- Analyzing Deviations and Outliers: If a manufacturing process produces outliers, such as batches with suboptimal quality, histograms can quickly identify these deviations. This is crucial for maintaining regulatory compliance and ensuring that defective products do not reach the market. Histograms allow quality control teams to focus on the causes of variability and adjust the manufacturing process as needed.
By using histogram analysis, manufacturers can ensure that their processes remain within controlled limits, preventing costly errors and maintaining product consistency. This not only safeguards patient safety but also enhances the company’s ability to meet regulatory standards.
6. Histogram Analysis in Regulatory Compliance and Safety
Pharmaceutical companies are subject to stringent regulatory requirements, particularly when it comes to ensuring drug safety and efficacy. Histogram analysis is a valuable tool for meeting these regulatory demands, offering clear, easily interpretable data that can be used in submissions to agencies like the FDA or EMA.
- FDA Requirements for Data Analysis: The FDA often requires the submission of extensive data showing the safety and efficacy of new drugs. Histograms can be included in regulatory submissions to visualize the distribution of key data points, such as the range of patient responses or the frequency of adverse events. This helps regulators assess whether the drug performs consistently across the target population.
- Adverse Event Frequency Analysis: Regulators pay close attention to the frequency and severity of adverse events. Histogram analysis allows companies to visualize AE data clearly, showing how often different AEs occur and whether certain events are more common in specific subgroups of patients. This aids in determining the risk profile of a drug, which is essential for regulatory approval.
- Consistent Data Reporting for Safety Standards: Consistency in data reporting is crucial for regulatory compliance. Histograms help pharmaceutical companies present their data in a standardized format that regulators can easily interpret, ensuring that the safety and efficacy of the drug are communicated clearly and accurately.
By incorporating histogram analysis into regulatory submissions, pharmaceutical companies can provide a compelling visual representation of their data, supporting their case for drug approval.
7. Challenges in Histogram Analysis and Solutions
While histograms are a powerful tool for data analysis, they are not without challenges, particularly in the context of the pharmaceutical and biopharma industries.
- Handling Large Datasets: The sheer volume of data generated during drug development and manufacturing can be overwhelming. In some cases, histograms can become cluttered and difficult to interpret when dealing with very large datasets. To address this, advanced software tools can be used to segment data or dynamically adjust bin sizes, making histograms more manageable.
- Data Integrity and Quality Issues: The quality of data used to create histograms is critical. Inaccurate or incomplete data can lead to misleading conclusions. Ensuring that all data is thoroughly vetted before it is used for histogram analysis is essential for producing reliable insights.
- Interpreting Complex or Overlapping Distributions: Some datasets may have complex or overlapping distributions that are difficult to interpret using simple histograms. In such cases, more sophisticated analysis techniques, such as kernel density estimation or multi-modal distribution analysis, may be required to supplement histogram insights.
By recognizing these challenges and adopting best practices, pharmaceutical and biopharma companies can maximize the utility of histograms in their data analysis processes.
8. Future Trends in Histogram Analysis in Pharma and Biopharma
As technology continues to evolve, so too will the tools and methods used for histogram analysis in the pharmaceutical and biopharma industries. Several emerging trends are likely to shape the future of data analysis in these sectors:
- Machine Learning and AI-driven Data Analysis: AI and machine learning are becoming increasingly important in drug discovery and clinical trials. These technologies can automatically generate histograms from large datasets and even identify patterns that might not be immediately obvious to human analysts.
- Real-Time Histogram Monitoring in Manufacturing: Advances in sensor technology and real-time data analytics will allow manufacturers to generate histograms in real time, enabling them to monitor production processes more closely and react to deviations immediately.
- Integration with Big Data and Predictive Analytics: As the pharmaceutical industry continues to generate larger datasets, integrating histogram analysis with big data platforms and predictive analytics tools will become increasingly common. This will help companies gain deeper insights into drug development and manufacturing processes.
By staying at the forefront of these technological advancements, the pharmaceutical and biopharma industries will be able to use histogram analysis more effectively to drive innovation and maintain quality and compliance.
Conclusion
Histogram analysis is a vital tool across all stages of pharmaceutical and biopharma development, from drug discovery and clinical trials to manufacturing and regulatory compliance. As the industry continues to evolve, adopting advanced technologies and addressing challenges in data interpretation will be key to leveraging the full potential of histogram analysis. By doing so, companies can enhance their ability to make data-driven decisions, ensuring the safety, efficacy, and quality of their products.