Creating Tomorrow: The Rise Of Generative Ai In Art, Design, And Beyond
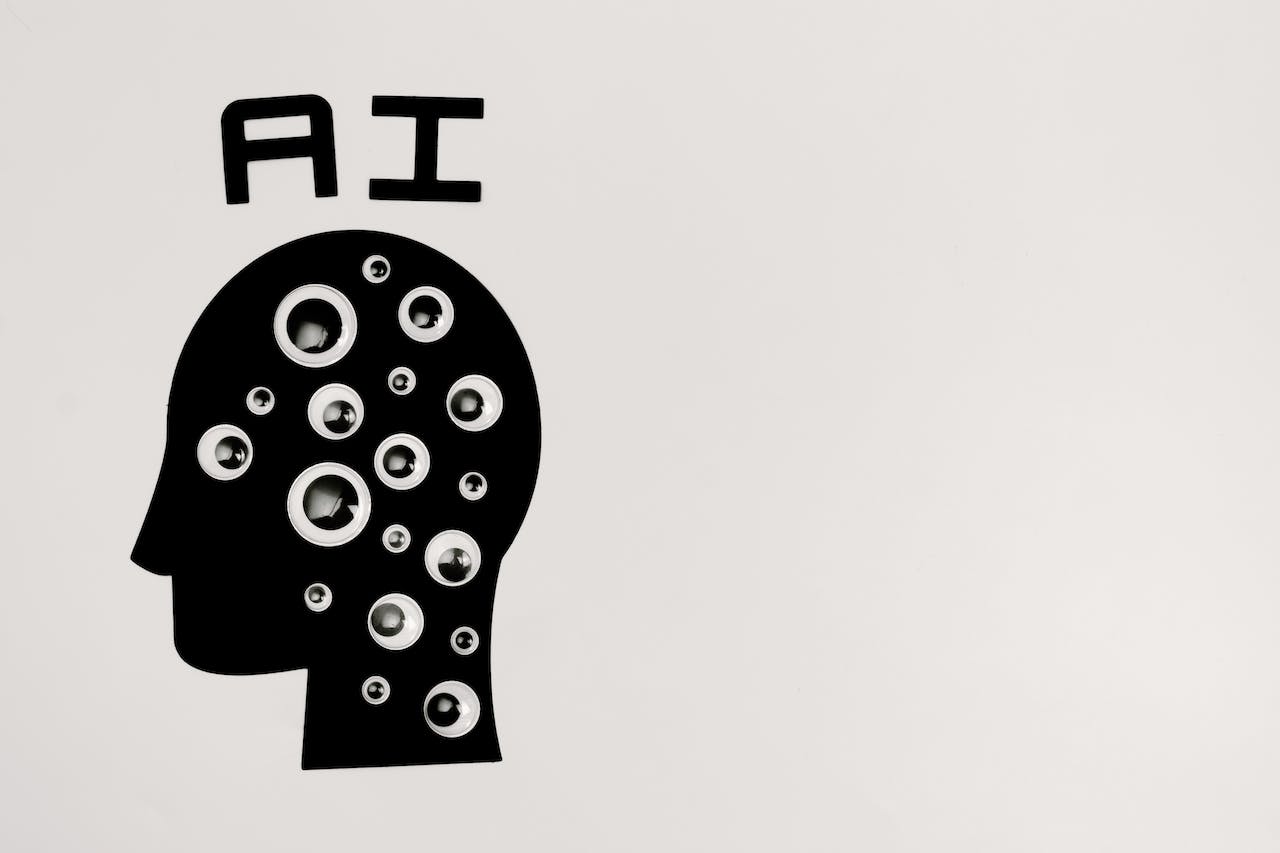
Introduction:
In the ever-evolving realm of artificial intelligence, one of the most fascinating and transformative advancements is Generative AI. This cutting-edge technology is revolutionizing how machines understand, interpret, and create content. In this comprehensive exploration, we will demystify Generative AI, diving into its definition, workings, and the profound impact it has on various industries.
What is Generative AI?
Generative AI (gen ai) is a subset of artificial intelligence that focuses on enabling machines to generate content autonomously. Unlike traditional AI models that are trained to recognize patterns in existing data, generative models have the remarkable ability to create entirely new content, whether it be images, text, or even music.
Types of Generative AI Models:
-
Generative Adversarial Networks (GANs): GANs are a popular class of generative models introduced by Ian Goodfellow and his colleagues in 2014. GANs consist of two neural networks - a generator and a discriminator - engaged in a constant game of one-upmanship. The generator creates content, and the discriminator assesses its authenticity. This iterative process results in the generation of highly realistic and diverse content.
-
Variational Autoencoders (VAEs): VAEs are another type of generative model that focuses on learning the underlying structure of the input data. By understanding the distribution of the data, VAEs can then generate new samples that resemble the original input. VAEs are particularly adept at producing variations of input data, making them valuable in creative applications.
Generative AI Meaning
Generative AI, short for Generative Artificial Intelligence, refers to a category of artificial intelligence systems and models designed to generate new content autonomously. Unlike traditional AI models that are mainly focused on recognizing patterns in existing data, generative models have the remarkable ability to create entirely new content, whether it be images, text, music, or other forms of data.
The key characteristic of generative AI lies in its creativity and ability to produce novel output that wasn't explicitly present in its training data. These systems learn the underlying patterns and structures of the data they are exposed to during the training phase, and once trained, they can generate content that exhibits similar characteristics.
Generative AI models can take various forms, with notable examples including Generative Adversarial Networks (GANs) and Variational Autoencoders (VAEs). GANs, for instance, consist of two neural networks – a generator and a discriminator – engaged in a constant feedback loop. The generator creates content, and the discriminator evaluates its authenticity. This iterative process results in the generation of highly realistic and diverse content.
How Generative AI Works:
Understanding the inner workings of Generative AI involves delving into the functioning of the specific models it employs.
1. Training Phase:
Generative AI models begin their journey in the training phase. During this stage, the model is fed vast amounts of data to learn patterns, styles, and structures. Whether it's images, text, or other forms of data, the model dissects and comprehends the underlying features that define the input.
2. Generative Process:
Once trained, the generative model enters the phase where it autonomously produces new content. For example, in GANs, the generator network starts creating data, while the discriminator evaluates its authenticity. This iterative process refines the generator's ability to create content that is increasingly indistinguishable from real data.
3. Feedback Loop:
The interplay between the generator and discriminator creates a feedback loop. The generator strives to produce content that is more convincing, and the discriminator adapts to discern increasingly subtle nuances. This dynamic equilibrium results in the generation of content that often surprises with its realism and creativity.
Here Are Some Notable Generative AI Examples:
-
DeepArt and Neural Style Transfer: Platforms like DeepArt use Generative AI to apply the style of famous artworks to users' photos. Neural Style Transfer, a technique utilizing Generative AI, enables the creation of visually stunning and artistically styled images.
-
OpenAI's GPT-3 for Text Generation: OpenAI's GPT-3 (Generative Pre-trained Transformer 3) is a state-of-the-art language model that excels in natural language understanding and generation. It can generate coherent and contextually relevant text across a wide range of topics.
-
GANs in Image Synthesis: Generative Adversarial Networks (GANs) are widely employed for image synthesis. Artists and designers leverage GANs to generate realistic faces, landscapes, and even entirely fictional scenarios. This technology has applications in the gaming industry, art, and virtual world creation.
-
Google's DeepDream: DeepDream, developed by Google, is an example of Generative AI applied to image recognition. It generates dreamlike images by enhancing and modifying patterns recognized by neural networks, resulting in visually striking and surreal creations.
-
Artbreeder for Visual Exploration: Artbreeder is an interactive platform that allows users to blend and manipulate images using Generative AI. Users can experiment with different features, creating unique and often mesmerizing visuals by exploring the latent space of images.
-
Magenta Studio for Music Composition: Magenta, a research project by Google, focuses on using Generative AI for music and art. Magenta Studio offers tools for musicians and artists to explore and create music compositions, leveraging machine learning algorithms for creative expression.
-
DALL-E by OpenAI: DALL-E is another creation by OpenAI, demonstrating Generative AI's prowess in image generation. This model can generate diverse and imaginative images based on textual descriptions, showcasing its ability to understand and translate textual prompts into visual content.
-
AI Dungeon for Interactive Storytelling: AI Dungeon utilizes GPT-3 to create dynamic and interactive storytelling experiences. Users can input prompts, and the model generates detailed and contextually relevant storylines, adapting to the user's input in real-time.
-
Deepfake Technology: While controversial, deepfake technology is an application of Generative AI that involves replacing one person's likeness in video footage with another's. This has implications in the entertainment industry, but it also raises ethical concerns about the potential misuse of such technology.
-
Generative AI in Video Game Design: In the gaming industry, Generative AI is used to create realistic environments, characters, and even dialogues. Procedural content generation powered by generative models allows for the dynamic creation of game elements, enhancing the gaming experience.
Applications of Generative AI:
Generative AI has transcended the realm of theoretical concepts and found practical applications across diverse industries.
1. Art and Creativity:
In the realm of art, Generative AI has emerged as a powerful tool for creating unique and visually stunning pieces. Artists and designers leverage generative models to explore new styles, generate digital art, and push the boundaries of creativity.
2. Content Generation:
From text to images, Generative AI is transforming content creation. Automated content generators can produce articles, stories, or even generate images based on specific themes or input parameters. This has implications for industries such as journalism, marketing, and graphic design.
3. Medical Imaging:
In the medical field, Generative AI is making strides in generating realistic medical images. This has implications for medical training, simulations, and even in improving the quality of medical imaging through the creation of synthetic data.
4. Game Development:
The gaming industry has embraced Generative AI for creating immersive and dynamic gaming environments. Procedural content generation, powered by generative models, can generate realistic landscapes, characters, and even storylines.
5. Fashion and Design:
In the world of fashion, Generative AI is being employed to design clothing, generate new styles, and even predict fashion trends. This not only streamlines the design process but also introduces novel and avant-garde concepts.
The Ethical Dimensions:
While the capabilities of Generative AI are awe-inspiring, they also raise ethical considerations and challenges.
1. Deepfakes and Misuse:
The ability of Generative AI to create realistic content has given rise to concerns about deepfakes – manipulated content that can be used to deceive or spread misinformation. Addressing the misuse of generative models is a crucial ethical consideration.
2. Bias in Data:
The models used in Generative AI are only as good as the data they are trained on. If the training data contains biases, the generated content may also reflect these biases. Ensuring fairness and mitigating bias in generative models is an ongoing challenge.
3. Privacy Concerns:
Generative AI's capacity to create realistic faces or personas raises privacy concerns. Striking a balance between the generation of authentic content and respecting individuals' privacy is an ethical imperative.
Future Trends and Innovations:
As Generative AI continues to evolve, several trends and innovations are shaping its trajectory.
1. Customization and Personalization:
The future of Generative AI lies in its ability to tailor content to individual preferences. Whether it's personalized content recommendations or customized digital avatars, the emphasis is on creating unique experiences for users.
2. Cross-Domain Creativity:
Generative models are increasingly demonstrating the ability to create content across different domains. This cross-domain creativity holds promise for applications that require the synthesis of diverse types of data.
3. Explainable AI:
Addressing the "black box" nature of deep learning models is a growing area of research. Ensuring that generative models provide explanations for their outputs is crucial for building trust and understanding their decisions.
Conclusion:
In conclusion, Generative AI represents a paradigm shift in how we approach creativity and content generation. From transforming artistic expression to revolutionizing content creation in various industries, the impact of generative models is profound. As we navigate the ethical considerations and embrace the innovations shaping the future, Generative AI stands as a testament to the limitless possibilities of artificial intelligence. In a world where machines not only learn from data but create new and imaginative content, we find ourselves at the frontier of a technological renaissance. The journey of Generative AI is a testament to the uncharted territories that await exploration in the ever-expanding landscape of artificial intelligence.