Can Ai Solve The Clinical Data Problem?
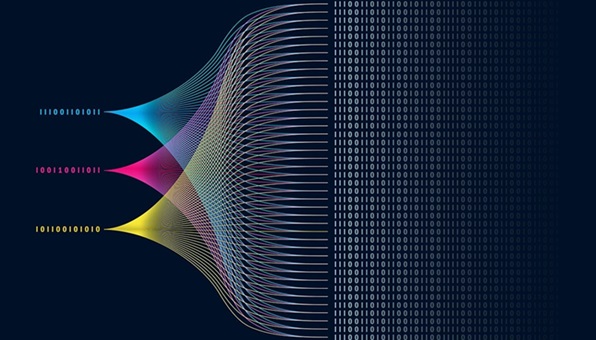
Introduction
The healthcare industry is facing an unprecedented data explosion. Every day, massive amounts of clinical data are generated from various sources, including electronic health records (EHRs), medical imaging, lab results, and wearable devices. While this wealth of information holds the potential to transform patient care and outcomes, managing, analyzing, and utilizing this data effectively remains a significant challenge. The complexity of clinical data, combined with issues of data quality, integration, and privacy, has created what is often referred to as the "clinical data problem."
Artificial intelligence (AI) offers promising solutions to these challenges. With its ability to process vast amounts of data quickly and accurately, AI can help healthcare providers make sense of clinical data, leading to improved patient outcomes, streamlined operations, and reduced costs. But can AI truly solve the clinical data problem? This article explores the potential of AI to revolutionize clinical data management and the obstacles that must be overcome to realize this vision.
Understanding the Clinical Data Problem
Clinical data encompasses a wide range of information generated during the course of patient care. This data is crucial for diagnosing conditions, planning treatments, and conducting medical research. However, several factors make managing clinical data particularly challenging:
- Types of Clinical Data and Their Sources
Clinical data comes in various forms, including structured data (like lab results and medication records) and unstructured data (such as physician notes and medical imaging). It is collected from numerous sources, including EHRs, medical devices, patient registries, and research databases. - Challenges in Managing Clinical Data
Managing clinical data effectively requires overcoming several challenges:
- Data Volume and Variety: The sheer volume of clinical data generated daily can be overwhelming. In addition to traditional medical records, new data streams from wearable devices, genomics, and patient-reported outcomes add to the complexity.
- Data Quality and Consistency: Inaccurate, incomplete, or inconsistent data can lead to incorrect diagnoses and treatment plans. Variability in how data is collected, recorded, and coded also complicates data quality management.
- Data Interoperability: Different healthcare systems often use incompatible data formats and standards, making it difficult to share and integrate data across platforms. Lack of interoperability hampers the seamless flow of information between providers, affecting care coordination and patient outcomes.
- Privacy and Security Concerns: Clinical data contains sensitive patient information that must be protected from unauthorized access and breaches. Ensuring data privacy and security is a major challenge, especially when handling large datasets across multiple platforms.
How AI Can Address Clinical Data Challenges
AI technologies, including machine learning, natural language processing (NLP), and computer vision, offer powerful tools to address the complexities of clinical data management. Here’s how AI can help:
- Data Integration and Interoperability
AI can facilitate data integration and interoperability by automatically mapping and converting data from different formats into a unified structure. Machine learning algorithms can identify patterns and correlations in disparate datasets, helping to standardize data and make it more compatible across various systems. For example, AI can help integrate EHR data with imaging results and genomic information, providing a comprehensive view of a patient’s health. - Data Quality and Standardization
AI can significantly enhance data quality by identifying and correcting errors, filling in missing information, and ensuring consistency across records. NLP algorithms can analyze unstructured data, such as physician notes and discharge summaries, extracting valuable information and converting it into structured formats. This helps create more accurate and complete patient records, which are essential for effective care delivery. - Data Analysis and Insights
AI excels at analyzing large datasets to uncover insights that might be missed by human analysts. Machine learning algorithms can detect patterns and trends in clinical data, helping healthcare providers identify risk factors, predict disease progression, and personalize treatment plans. AI-powered analytics can also support population health management by analyzing trends and outcomes across large patient groups. - Predictive Analytics and Decision Support
AI can enhance clinical decision-making by providing predictive analytics and decision support tools. For instance, machine learning models can predict patient outcomes based on historical data, helping clinicians identify high-risk patients and intervene early. AI can also assist in diagnostic decision-making by analyzing imaging data or suggesting possible diagnoses based on a patient’s symptoms and medical history. - Automation of Administrative Tasks
AI can automate time-consuming administrative tasks, such as data entry, coding, and billing, freeing up healthcare professionals to focus on patient care. Automation can reduce errors and improve efficiency in healthcare operations, leading to better resource allocation and cost savings.
Case Studies: AI Solving Clinical Data Problems
Several organizations have successfully implemented AI solutions to tackle clinical data challenges. Here are a few examples:
- Mayo Clinic: Enhancing Diagnostics with AI
The Mayo Clinic has developed an AI-based algorithm to interpret ECG signals, identifying patients at risk for atrial fibrillation (AFib) even before they show symptoms. By analyzing vast amounts of ECG data, the AI model can detect subtle patterns indicative of AFib, allowing for early intervention and preventing serious complications like stroke. - Google Health: Improving Cancer Detection
Google Health has developed an AI system that can analyze mammograms more accurately than radiologists. By training the AI on millions of mammogram images, Google Health's model can detect breast cancer with fewer false positives and negatives, improving early detection rates and reducing the need for unnecessary biopsies. - Johns Hopkins Medicine: Reducing Sepsis Mortality with AI
Johns Hopkins Medicine implemented an AI-based early warning system called "Sepsis Watch," which continuously monitors patient data to detect early signs of sepsis. The system alerts clinicians to high-risk patients, enabling timely interventions and reducing sepsis-related mortality rates.
Limitations and Challenges of Using AI in Clinical Data
While AI holds great promise for solving clinical data problems, several challenges and limitations need to be addressed:
- Technical Limitations
AI systems require large amounts of high-quality data to train accurate models. In many cases, clinical data is fragmented, inconsistent, or incomplete, making it difficult to develop reliable AI solutions. Additionally, AI models can be prone to bias if trained on unrepresentative datasets, leading to inaccurate predictions and potential harm to patients. - Ethical and Regulatory Concerns
The use of AI in healthcare raises ethical questions about transparency, accountability, and fairness. Ensuring that AI models are explainable and that their decision-making processes are transparent is crucial for building trust among healthcare providers and patients. Furthermore, regulatory bodies must establish guidelines and standards for the safe and effective use of AI in clinical settings. - Data Privacy and Security Risks
Handling large volumes of sensitive clinical data increases the risk of privacy breaches and data security incidents. AI systems must comply with stringent data protection regulations, such as the Health Insurance Portability and Accountability Act (HIPAA) in the United States and the General Data Protection Regulation (GDPR) in the European Union. Implementing robust security measures and encryption techniques is essential to safeguard patient data.
The Future of AI in Solving Clinical Data Problems
The future of AI in healthcare is bright, with ongoing advancements in technology and increasing adoption across the industry. Here are some emerging trends and innovations:
- Federated Learning
Federated learning is an AI approach that enables machine learning models to be trained across multiple decentralized datasets without transferring the data to a central server. This technique can enhance data privacy and security while allowing AI models to learn from diverse datasets, improving accuracy and generalizability. - Explainable AI
As AI becomes more integrated into clinical decision-making, there is a growing demand for explainable AI models that can provide clear, understandable explanations for their predictions. Explainable AI helps build trust among clinicians and patients and ensures that AI-driven decisions are transparent and accountable. - Integration with Electronic Health Records (EHRs)
EHR systems are increasingly incorporating AI capabilities to enhance data management, improve clinical workflows, and support decision-making. By integrating AI tools directly into EHRs, healthcare providers can access real-time insights and recommendations, leading to more informed and timely care. - AI for Precision Medicine
AI is playing a pivotal role in advancing precision medicine, which tailors treatments to individual patients based on their genetic, environmental, and lifestyle factors. AI algorithms can analyze complex datasets, including genomic data, to identify personalized treatment options and predict patient responses to therapies.
Conclusion
AI has the potential to revolutionize the way clinical data is managed, analyzed, and utilized in healthcare. By addressing key challenges such as data integration, quality, and interoperability, AI can unlock valuable insights that improve patient outcomes, streamline operations, and reduce costs. However, realizing the full potential of AI in solving clinical data problems requires overcoming technical limitations, ensuring ethical use, and safeguarding data privacy and security.
As the healthcare industry continues to evolve, the integration of AI into clinical workflows will become increasingly important. By embracing AI technologies and fostering a culture of innovation, healthcare organizations can harness the power of clinical data to drive better care, enhance patient experiences, and ultimately, improve population health.
Visit Our Service:https://likeways.co.in/resume-build-and-review/