Big Data In The Pharmaceutical Industry
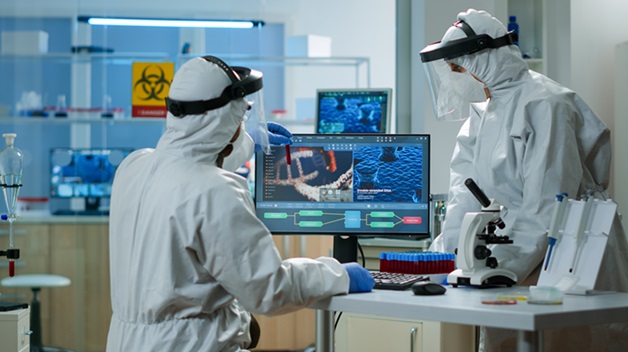
The pharmaceutical industry is undergoing a significant transformation, driven largely by the integration of big data analytics. Big data has emerged as a powerful tool for pharmaceutical companies, enabling them to improve drug development processes, optimize clinical trials, enhance patient outcomes, and reduce costs. This blog will explore the role of big data in the pharmaceutical industry, its applications, benefits, challenges, and future prospects.
1. Introduction to Big Data in the Pharmaceutical Industry
Big data refers to the vast volumes of structured and unstructured data that are generated, collected, and analyzed across various industries. In the pharmaceutical sector, big data encompasses a wide range of information sources, including clinical trial data, electronic health records (EHRs), genomic data, patient feedback, social media interactions, and real-world evidence. The sheer volume, variety, and velocity of data available today present both opportunities and challenges for pharmaceutical companies.
The integration of big data into the pharmaceutical industry marks a paradigm shift in how drugs are developed, tested, and brought to market. By leveraging advanced analytics and machine learning algorithms, pharmaceutical companies can gain deeper insights into disease mechanisms, identify potential drug targets, predict patient responses, and personalize treatments. This not only accelerates the drug development process but also improves the safety and efficacy of new therapies.
2. Applications of Big Data in the Pharmaceutical Industry
Big data has numerous applications in the pharmaceutical industry, spanning across various stages of the drug development lifecycle:
Drug Discovery and Development: Big data analytics plays a crucial role in drug discovery by identifying potential drug candidates and predicting their effectiveness. By analyzing large datasets from genomic research, chemical libraries, and biological studies, researchers can identify new drug targets and develop more effective therapies. Machine learning algorithms can also predict the success of a drug candidate based on its chemical properties and biological activity, reducing the time and cost associated with traditional drug discovery methods.
Clinical Trials: Clinical trials are a critical component of the drug development process, but they are often time-consuming, expensive, and prone to delays. Big data can streamline clinical trials by optimizing patient recruitment, identifying suitable trial sites, and monitoring patient adherence. By analyzing EHRs and real-world data, pharmaceutical companies can identify patients who meet specific inclusion criteria, reducing the time and cost of patient recruitment. Additionally, big data can be used to monitor patient outcomes in real-time, enabling researchers to make data-driven decisions and adjust trial protocols as needed.
Personalized Medicine: Personalized medicine aims to tailor treatments to individual patients based on their genetic makeup, lifestyle, and other factors. Big data plays a crucial role in personalized medicine by analyzing genomic data, EHRs, and other data sources to identify biomarkers and predict patient responses to specific treatments. This enables pharmaceutical companies to develop targeted therapies that are more effective and have fewer side effects, improving patient outcomes and reducing healthcare costs.
Drug Safety and Pharmacovigilance: Ensuring the safety of drugs is a top priority for pharmaceutical companies and regulatory agencies. Big data analytics can enhance drug safety by identifying adverse drug reactions (ADRs) and monitoring the safety of drugs post-market. By analyzing EHRs, social media data, and other sources, pharmaceutical companies can detect potential safety signals and take corrective actions before a drug is widely distributed. This proactive approach to pharmacovigilance helps protect patient safety and minimize the risk of costly recalls or lawsuits.
Market Access and Commercialization: Big data can also be used to inform market access strategies and optimize the commercialization of new drugs. By analyzing data on patient demographics, prescribing patterns, and healthcare utilization, pharmaceutical companies can identify market opportunities, target specific patient populations, and develop effective marketing campaigns. This data-driven approach to commercialization helps ensure that new therapies reach the right patients and achieve maximum impact.
3. Benefits of Big Data in the Pharmaceutical Industry
The integration of big data into the pharmaceutical industry offers several key benefits:
Accelerated Drug Development: By leveraging big data analytics, pharmaceutical companies can streamline the drug development process and bring new therapies to market faster. This not only reduces costs but also allows patients to access life-saving treatments sooner.
Improved Clinical Trial Efficiency: Big data can enhance the efficiency of clinical trials by optimizing patient recruitment, identifying suitable trial sites, and monitoring patient adherence. This helps reduce the time and cost associated with clinical trials, increasing the likelihood of successful outcomes.
Enhanced Drug Safety: Big data analytics can improve drug safety by identifying adverse drug reactions and monitoring the safety of drugs post-market. This proactive approach to pharmacovigilance helps protect patient safety and reduce the risk of costly recalls or lawsuits.
Personalized Medicine: Big data enables the development of personalized therapies tailored to individual patients based on their genetic makeup, lifestyle, and other factors. This not only improves patient outcomes but also reduces healthcare costs by ensuring that patients receive the most effective treatments.
Informed Decision-Making: By analyzing large datasets from various sources, pharmaceutical companies can gain deeper insights into disease mechanisms, drug targets, patient outcomes, and market trends. This data-driven approach to decision-making helps optimize drug development processes, improve patient outcomes, and maximize commercial success.
4. Challenges of Big Data in the Pharmaceutical Industry
While big data offers numerous benefits, its integration into the pharmaceutical industry also presents several challenges:
Data Quality and Integration: One of the biggest challenges in big data analytics is ensuring the quality and integrity of data. Pharmaceutical companies often rely on data from multiple sources, including EHRs, genomic data, social media, and real-world evidence. These data sources may have different formats, structures, and levels of accuracy, making it difficult to integrate and analyze them effectively. Ensuring data quality and consistency is essential for accurate analysis and reliable insights.
Data Privacy and Security: The use of big data in the pharmaceutical industry raises significant privacy and security concerns. Patient data is highly sensitive and must be protected to ensure patient confidentiality and comply with regulations such as the Health Insurance Portability and Accountability Act (HIPAA) and the General Data Protection Regulation (GDPR). Pharmaceutical companies must implement robust data security measures to protect patient data from unauthorized access, breaches, and misuse.
Regulatory Compliance: The pharmaceutical industry is heavily regulated, and the use of big data analytics must comply with various regulatory requirements. Regulatory agencies such as the FDA and the European Medicines Agency (EMA) have specific guidelines for the use of real-world data and big data analytics in drug development and approval processes. Ensuring compliance with these regulations is essential for avoiding legal and financial penalties.
Data Silos and Fragmentation: Data silos and fragmentation can hinder the effective use of big data in the pharmaceutical industry. Data is often stored in separate systems and departments, making it difficult to access and analyze. Breaking down data silos and integrating data from different sources is essential for gaining a comprehensive view of the data and extracting meaningful insights.
Skill Gaps and Talent Shortages: The successful implementation of big data analytics in the pharmaceutical industry requires specialized skills and expertise in data science, machine learning, and biostatistics. However, there is a shortage of skilled professionals in these fields, making it challenging for pharmaceutical companies to build and maintain effective data analytics teams.
5. Future Prospects of Big Data in the Pharmaceutical Industry
The future of big data in the pharmaceutical industry is bright, with several emerging trends and technologies set to shape the industry in the coming years:
Artificial Intelligence and Machine Learning: Artificial intelligence (AI) and machine learning (ML) are poised to revolutionize the pharmaceutical industry by enabling more advanced data analytics and predictive modeling. AI and ML algorithms can analyze large datasets from various sources to identify patterns, predict patient responses, and optimize drug development processes. This will enable pharmaceutical companies to develop more effective therapies, reduce costs, and improve patient outcomes.
Real-World Evidence and Digital Health: Real-world evidence (RWE) and digital health technologies are becoming increasingly important in the pharmaceutical industry. RWE refers to data collected from real-world settings, such as EHRs, patient registries, and wearable devices, to evaluate the safety and effectiveness of treatments. By leveraging RWE and digital health technologies, pharmaceutical companies can gain deeper insights into patient outcomes and improve drug development processes.
Blockchain Technology: Blockchain technology has the potential to address some of the challenges associated with data privacy, security, and interoperability in the pharmaceutical industry. By providing a secure, decentralized, and transparent way to store and share data, blockchain can enhance data integrity, protect patient privacy, and facilitate data sharing across different stakeholders.
Collaborative Research and Data Sharing: Collaborative research and data sharing are becoming increasingly important in the pharmaceutical industry. By sharing data and resources, pharmaceutical companies can accelerate drug development processes, reduce costs, and improve patient outcomes. Initiatives such as the All of Us Research Program and the Global Alliance for Genomics and Health (GA4GH) are examples of collaborative efforts to leverage big data for medical research.
Personalized Medicine and Genomics: The growing focus on personalized medicine and genomics is driving the demand for big data analytics in the pharmaceutical industry. By analyzing genomic data and other patient-specific information, pharmaceutical companies can develop targeted therapies that are more effective and have fewer side effects. This trend is expected to continue in the coming years, with big data playing a central role in the development of personalized treatments.
6. Conclusion
Big data is revolutionizing the pharmaceutical industry, offering new opportunities to improve drug development processes, enhance patient outcomes, and reduce costs. By leveraging advanced analytics and machine learning algorithms, pharmaceutical companies can gain deeper insights into disease mechanisms, identify potential drug targets, predict patient responses, and personalize treatments. However, the integration of big data also presents several challenges, including data quality, privacy, security, and regulatory compliance. To fully realize the potential of big data, pharmaceutical companies must invest in the necessary infrastructure, talent, and technologies to effectively
Visit our Service:https://likeways.co.in/resume-build-and-review/